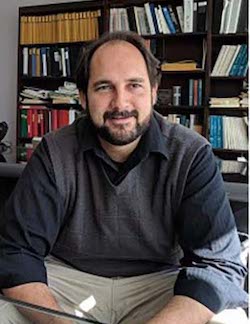
Expertise
- Computational Physics
- Electronic and Thermal Transport of Materials
- Mathematical Modeling
- Chaos and Nonlinear Dynamics
Brief Biography
Dr. Nicholas A. Mecholsky received his Ph.D. in Physics from the University of Maryland, College Park in 2010. He held a postdoctoral position at the Catholic University of America in 2011 and became a Vitreous State Lab Scientist in 2013. He is a Research Associate Professor in Catholic University of America’s Department of Physics.
Dr. Nicholas Mecholsky is an interdisciplinary computational and theoretical scientist in the areas of condensed matter research, nonlinear dynamics and chaos, and machine learning and data science. His research areas include nuclear waste glass formation, nuclear physics, machine learning, materials science, classical mechanics, electronic band structure, geodesy of wormholes, vision research, and game theory. In 2018 Dr. Mecholsky received Wolfram Research’s Innovator Award in a ceremony at the Wolfram Technology Conference. Dr. Mecholsky has taught university lecture courses for physics majors, engineers, interdisciplinary students, and graduate students including Introductory Physics, Honor’s Physics, Computational Physics, and Thermal Physics and Thermostatistics.
Research Interests
Dr. Mecholsky has a wide range of interests in computational physics and mathematical modeling.
Computational physics and mathematical modeling are important tools used by physicists to study a wide range of physical phenomena. Computational physics involves the use of computer simulations and other numerical methods to solve complex physical problems that cannot be easily studied using traditional analytical techniques. This approach allows physicists to study phenomena that are too complex, too large, or too small to be studied directly, and it can provide insight into the underlying physical processes that govern the behavior of systems.
Mathematical modeling, on the other hand, involves the use of mathematical equations and concepts to describe and understand physical systems. This approach allows physicists to develop predictive models that can be used to understand the behavior of systems under different conditions, and it can provide a framework for making quantitative predictions about the behavior of physical systems.
Together, computational physics and mathematical modeling are powerful tools that are used by physicists to study a wide range of phenomena, from the behavior of subatomic particles to the evolution of the universe as a whole. These approaches are essential for advancing our understanding of the physical world, and they play a vital role in many areas of science and technology.
Some of his active areas of research include:
Models for Separation of Labeled Data in High Dimensional Systems:
(with Konstantin Gilbo, Ian L. Pegg) Support Vector Machines (SVMs) and other machine learning algorithms can be used to separate complicated labeled datasets. These algorithms can learn patterns and relationships within the data, and then use that information to accurately classify the data into different groups or categories based on initial properties, such as the composition of nuclear waste glass. This can be useful for a variety of tasks, such predicting the properties of new types of glass based on composition or classifying glass samples based on their suitability for disposal. By using machine learning algorithms, researchers can more easily and accurately process and analyze large datasets, which can help to improve our understanding of nuclear waste glass and its behavior under different conditions as well as predicting which compositions will be suitable for disposal and what additives will be needed for processing.
In this research, the collaboration uses the VSL Nepheline dataset to determine if Nepheline will crystallize during canister cooling based on the input composition. Additionally, new methods in high dimensional reduction of complex data are under investigation.
Electron Transport in Semiconductor and Metals: (with Helen McDonough (assisted by past REU students Ashraful Haque (2021) and Virginia Jarvis (2022)) Electron transport is important in semiconductors and metals because it plays a crucial role in the functioning of these materials. In semiconductors, electron transport is responsible for the movement of electrons from the valence band, where they are bound to atoms, to the conduction band, where they are free to move and conduct electricity. This movement of electrons allows semiconductors to be used in a wide range of electronic devices, such as transistors and solar panels. In metals, electron transport is responsible for the flow of electrical current through the material. This makes metals an essential component in the construction of many electrical devices, such as wiring and circuit boards. In short, electron transport is a fundamental property of semiconductors and metals that makes them useful for a wide range of applications.
In this research the collaboration developed a Monte Carlo transport code following the seminal paper by Jacoboni and Reggiani (Jacoboni, C., & Reggiani, L. (1983). The Monte Carlo method for the solution of charge transport in semiconductors with applications to covalent materials. Reviews of modern Physics, 55(3), 645.). Additionally, the m*2T code (with Marco Fornari and Luca Bonaldo), a comprehensive code to predict temperature and chemical potential variations on thermoelectric properties of materials, is being developed.
Drift Velocity in Systems with Elastic Scattering rules: (with Rachel Morin) Typical classical systems that eventually result in a meaningful drift velocity almost always include some process to lose energy (including a thermal bath or other non-conservative processes). In some simulations, it became clear that this may not be strictly a necessary condition for a drift velocity.
In this investigation, the collaboration is exploring different possible scenarios that lead to a drift velocity in classical systems without any inelastic mechanisms.
Reservoir Computing: (with Francesco Sorrentino, Chandra Pappu, Chad Nathe, Thomas Carrol, Lou Pecora) Reservoir computing is a type of computational model that is used for solving a wide range of problems, including machine learning, signal processing, and time series analysis. In a reservoir computing system, a large, interconnected network of simple processing units, known as a "reservoir," is used to perform the computation. The key advantage of this approach is that the reservoir can be trained to perform a wide range of tasks using a single, fixed set of weights, which makes it highly flexible and efficient. This makes reservoir computing an attractive approach for many applications, especially those that require the ability to quickly and easily adapt to changing conditions.
In this research the collaboration is looking at various impacts of the implementation of reservoir computers including the impact of noise and the possibility of applying control to the input to achieve a desired output.
Models for Crack Branching and Multifractal statistics of Crack Branching: (with John Mecholsky, Jr., Stephen Freiman, and Roman Mecholsky) Models for crack branching are important for a variety of reasons. First, understanding the behavior of cracks as they branch and spread is essential for predicting the strength and durability of materials. This information is critical for the design and construction of safe and reliable structures, such as bridges, buildings, and aircraft. Second, studying crack branching can provide insight into the underlying physical processes that govern the behavior of materials, which can help to improve our understanding of how materials respond to various types of stress and strain. Finally, models of crack branching can be used to develop new materials and manufacturing processes that are more resistant to cracking and other forms of failure.
Here the collaboration is interested in various aspects of crack branching in ring-on-ring glass disks. Particularly interesting is the fractal nature of the crack branching coefficient as well as the possibility of distilling the fracture pattern into simpler structural components.
Density Functional Theoretical Computation of the Raman Spectrum of Pyrolusite and other Manganese polymorphs: (with Luis Aguinaga Del Hierro and David McKeown) Density functional theory (DFT) is a powerful computational tool that is widely used in the study of materials. DFT is a mathematical approach that is used to describe the behavior of electrons in materials, and it is based on the concept that the total energy of a system of electrons can be expressed as a functional of the system's electron density. This allows researchers to study the electronic structure of materials at the atomic level, which is essential for understanding their physical and chemical properties. DFT is widely used in the field of materials science to predict the properties of new materials, to design materials with specific properties, and to study the behavior of materials under different conditions.
The collaboration would like to use DFT to predict and compute the Raman spectrum of particularly complicated manganese polymorphs to compare to experimental measurements done by David McKeown and Jeff Post.
The Effects of Warping on Electronic Band Structures: (with Lorenzo Resca) The electronic band structure of materials is an important concept in materials science because it provides insight into the behavior of electrons within a material. The band structure refers to the arrangement of energy levels that electrons occupy within a solid. It can be used to predict the electrical and thermal conductivity of a material, as well as its optical and magnetic properties. Understanding the electronic band structure is therefore essential for designing materials with specific properties and for optimizing their performance in various applications. Various physical properties can be derived from derivatives and features of the band structure.
In this research the consequences of non-analytic points in the electronic band structure of materials is explored.
Electrodynamic measurement and device design for velocity measurement in the Earth’s magnetic field: The Earth’s magnetic field is a resource that may be used in a number of ways to provide some indication of location and speed information in moving bodies. In this research the feasibility and mathematics necessary to design velocity measurement devices based on the Lorentz force law of electromagnetism is established and investigated.